Clinical day
The 2021 IEEE International Symposium on Biomedical Imaging program will include a “Clinical Day” on April 13th, 2021. This one-day symposium will feature recent developments in “AI-based approaches to biomedical image analysis in cancer”.
Machine learning has recently received a large amount of interest for the reconstruction and analysis of biomedical and pre-clinical imaging datasets. The ISBI Clinical Day aims to foster multi-disciplinary interactions among imaging scientists, clinicians, experimental researchers, and industry. The 2021 session will bridge between recent developments in multiscale biomedical imaging and healthcare application in cancer. It will include lectures, a panel discussion and ample time for interactions among participants.
Confirmed speakers
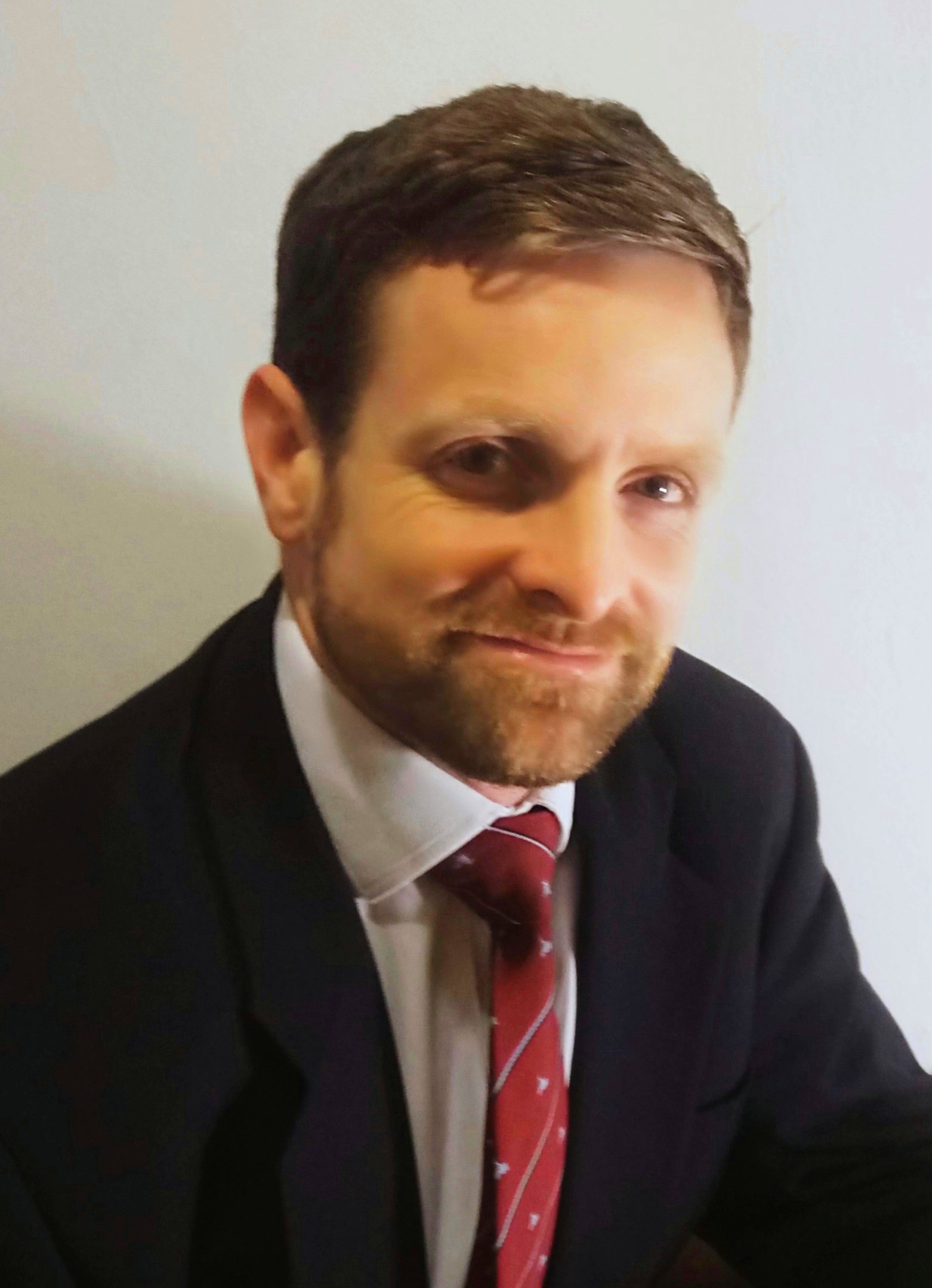
Biography
Thomas C Booth is a Senior Lecturer in Neuroimaging in the School of Biomedical Engineering & Imaging Sciences at King’s College London, UK. He is also an Honorary Neuroradiologist at King’s College Hospital, London, UK.
His interests include neuro-oncology research. He first started to enjoy machine learning applied to neuro-oncology during his PhD at the University of Cambridge, UK. Here, his focus was on brain tumour treatment response assessment using brain tumour MRI structural images – something he continues to research now as he is reminded continuously how important neuro-oncology diagnostics are when presenting patients at the neuro-oncology multi-disciplinary team meetings in a busy London teaching hospital. He is Chief Investigator on a multicentre machine learning neuro-oncology prospective study.
He sits on the National Cancer Research Institute Brain Tumour Committee and the Royal College of Radiologists Academic Committee. He was an awardee of the inaugural Royal College of Radiologists Outstanding Researcher Award.
Abstract: The ABC of translatable machine learning: AI, Biomarkers and the Clinic
The translation of algorithms derived from machine learning to the clinic is challenging. This lecture is designed to equip listeners with the knowledge to optimize study design. Biomarkers (diagnostic, prognostic, predictive, monitoring) will be defined. The steps in a radiomic study will be highlighted. Analytical and clinical validation will be explained. Limitations common to machine learning – which overlap with limitations in imaging research in general – will be described. Roadmaps and scoring systems for machine learning research will be outlined. Examples will focus on radiology in cancer.
This lecture will also provide the audience with an overview that will be useful for the rest of the clinical day.
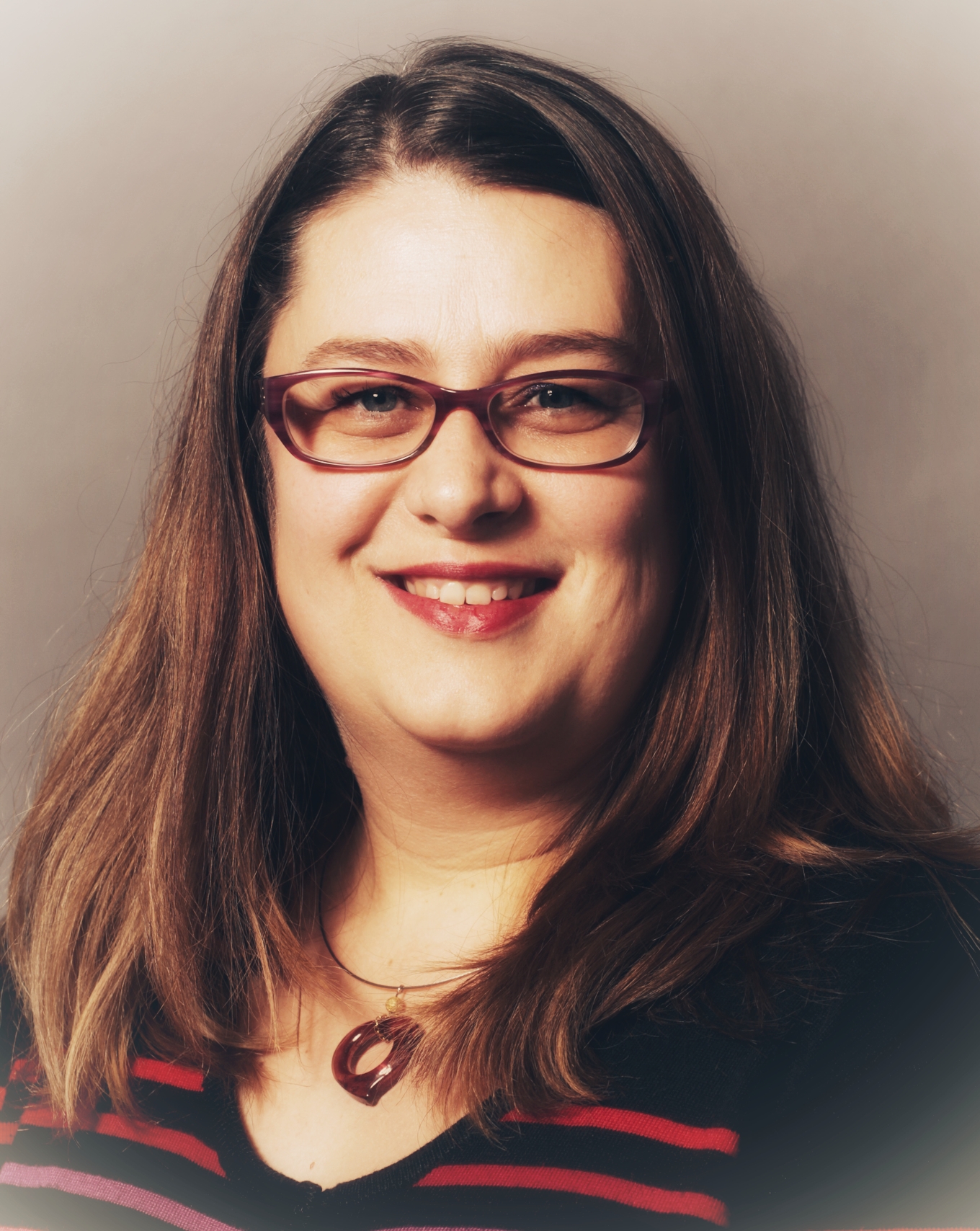
Laure Fournier
INSERM U970, Hôpital Européen Georges Pompidou, Paris, France
Biography
Dr Laure Fournier works as a Professor at the Hôpital Européen Georges Pompidou, in Paris, France. Her time is divided between clinical work on urogenital cancers, and imaging research in the Laboratoire de Recherche en Imagerie (INSERM U970).
She works on functional imaging, radiomics and big data, to extract quantitative parameters from images reflecting tumour physiology and biology, more specifically to define response to therapy, particularly for targeted therapies requiring new response criteria. She organises the curriculum on Artificial Intelligence for French radiology residents. She is a member of the Scientific Committee of the DRIM France IA national image database and is a medical imaging chair in the PRAIRIE interdisciplinary AI institute. She has been involved in outreach activities including round tables with clinicians and policy makers, meetings and interviews with patients and general public on the subject of patient databases, radiomics and AI.
Abstract: AI for medical imaging: what is missing to make it work?
AI is a promising field in medical imaging and has led to high expectations. However, some pitfalls and challenges have slowed down the development and implementation of these solutions, leading at worse to disappointment or distrust. We will go over the reasons for the difficulties in the practical implementation of AI solutions. At the training level, quality and quantity of data is the main challenge, including the relevance of the available data. At the validation level, there remain many questions on how these solutions should be validated to allow using them reliably and confidently. Finally, the implementation in the clinical workflow is a challenge in itself, both in technical and in clinical terms.
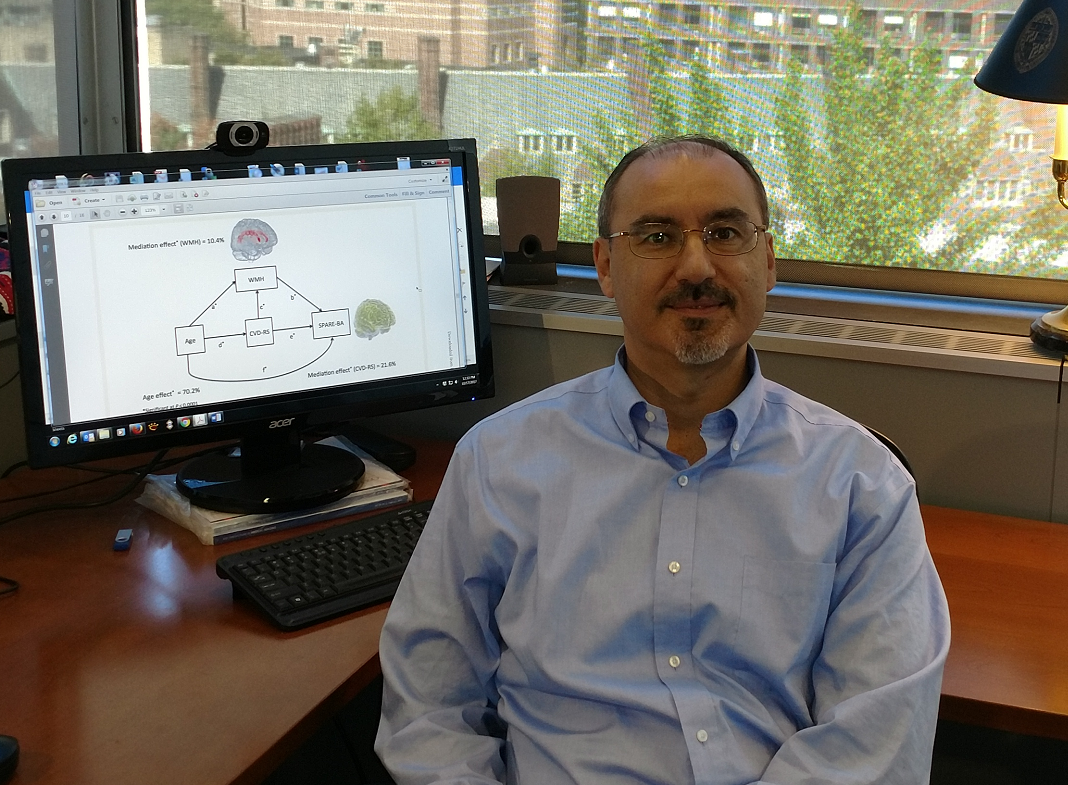
Biography
Christos Davatzikos is the Wallace T. Miller Sr. Professor of Radiology, with secondary appointment in Electrical and Systems Engineering and joint appointments with the Bioengineering and Applied Math graduate groups at Penn.
He received his undergraduate degree by the National Technical University of Athens, Greece, in 1989, and Ph.D. from Johns Hopkins University, in 1994. He joined the faculty at the Johns Hopkins School of Medicine as Assistant Professor (1995) and later Associate Professor (2001) of Radiology. In 2002 he moved to Penn to direct the Section for Biomedical Image Analysis, and in 2013 he established the Center for Biomedical Image Computing and Analytics.
His interests are in the field of imaging informatics. In the past 15 years he has focused on the application of machine learning and pattern analysis methods to medical imaging problems, including the fields of computational neuroscience and computational neuro-oncology. He has worked on aging, Alzheimer’s Disease, schizophrenia, brain development, and brain cancer.
Dr. Davatzikos is an IEEE and AIMBE Fellow, a Distinguished Investigator at the Academy of Radiology Research in the USA, and member of various editorial boards.
Abstract: Radiomics and Radiogenomics of glioblastoma
Machine learning methods have offered great promise for deriving imaging signatures of clinical and molecular measures of cancer. We present work focusing on glioblastoma, the most aggressive brain cancer, and show studies that have established radiomic and radiogenomic imaging signatures of clinical outcome as well as molecular characteristics of GBM. We also discuss implementation of these methods in the Cancer Imaging Phenomics Toolkit (CaPTk).
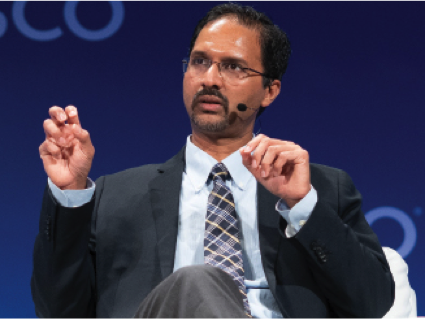
Anant Madabhushi
Case Western reserve University, Cleveland, USA
Biography
Anant Madabhushi, PhD, is the F. Alex Nason professor II of biomedical engineering at Case Western Reserve University (CWRU) in Cleveland and director of the university’s Center for Computational Imaging and Personalized Diagnostics (CCIPD). He is also a Research Scientist at the Louis Stokes Cleveland Veterans Administration (VA) Medical Center and has affiliate appointments both at University Hospitals and Cleveland Clinic. He holds secondary appointments in the departments of Biomedical Engineering, Urology, Radiology, Pathology, Radiation Oncology, Electrical Engineering & Computer Science and Gen Med Sciences at CWRU.
Madabhushi’s team at CCIPD is developing and applying novel Artificial Intelligence and machine learning approaches for the diagnosis, prognosis and prediction of therapy response for a variety of diseases including several different types of cancers, cardiovascular disease, kidney and eye disease. The Center is located in Cleveland’s unique medical ecosystem, an extensive clinical network within which it boasts numerous successful collaborations including with the Cleveland Clinic and the Cole Eye Institute, University Hospitals, the VA Louis Stokes Medical Center, MetroHealth, and the Case Comprehensive Cancer Center at CWRU.
Madabhushi has more than 100 patents either issued or pending in the areas of medical image analysis, computer-aided diagnosis, and computer vision, more than 50 of which are issued. He was responsible for 10 percent of all patents awarded to Case Western Reserve University in 2017, 2018 and 2019.
The author of more than 400 peer-reviewed journal articles and conference papers, Madabhushi is a sought after lecturer who has delivered more than 300 talks around the world. His efforts as a professor and researcher have gained international attention in the field of biomedical engineering, garnering him several awards. Most notably, Madabhushi is a Wallace H. Coulter Fellow, a fellow of the American Institute of Medical and Biomedical Engineering (AIMBE), and a Fellow of the Institute of Electrical and Electronics Engineers (IEEE). In 2015, he made Crain’s Cleveland Business magazine’s “Forty under 40” list. In 2019 and 2020, Madabhushi was named to The Pathologist’s Power List, a list of 100 most inspiring professionals in pathology and laboratory medicine. In 2020, he received the Diekhoff Award for Distinguished Graduate Student Mentoring at CWRU.
Madabhushi’s work on developing “smart computers for identifying lung cancer patients who will benefit from chemotherapy” was ranked as one of the top 10 medical breakthroughs of 2018 by Prevention Magazine. In 2019, Nature Magazine called him out as one of five scientists pursuing truly offbeat and innovative approaches in cancer research.
Madabhushi has secured more than $50 million in grant funding and co-founded two companies, Vascuvis Inc. (now Elucid Bioimaging) and IbRiS Inc., which was acquired by Inspirata in 2015. He has been involved in several sponsored research and industry partnerships with medical imaging and pharmaceutical companies. In addition, more than 15 technologies developed by Madabhushi’s team have been licensed.
Abstract: AI, Radiomics and Pathomics for Precision Oncology
Traditional biology generally looks at only a few aspects of an organism at a time and attempts to molecularly dissect diseases and study them part by part with the hope that the sum of knowledge of parts would help explain the operation of the whole. Rarely has this been a successful strategy to understand the causes and cures for complex diseases. The motivation for a systems based approach to disease understanding aims to understand how large numbers of interrelated health variables, gene expression profiling, its cellular architecture and microenvironment, as seen in its histological image features, its 3 dimensional tissue architecture and vascularization, as seen in dynamic contrast enhanced (DCE) MRI, and its metabolic features, as seen by Magnetic Resonance Spectroscopy (MRS) or Positron Emission Tomography (PET), result in emergence of definable phenotypes. At the Center for Computational Imaging and Personalized Diagnostics (CCIPD) at Case Western Reserve University, we have been developing computerized knowledge alignment, representation, and fusion tools for integrating and correlating heterogeneous biological data spanning different spatial and temporal scales, modalities, and functionalities. These tools include computerized feature analysis methods for extracting subvisual attributes for characterizing disease appearance and behavior on radiographic (radiomics) and digitized pathology images (pathomics). In this talk I will discuss the development work in CCIPD on new radiomic and pathomic approaches for capturing intra-tumoral heterogeneity and modeling tumor appearance. I will also focus my talk on how these radiomic and pathomic approaches can be applied to predicting disease outcome, recurrence, progression and response to therapy in the context of prostate, brain, rectal, oropharyngeal, and lung cancers. Additionally I will also discuss some recent work on looking at use of pathomics in the context of racial health disparity and creation of more precise and tailored prognostic and response prediction models.
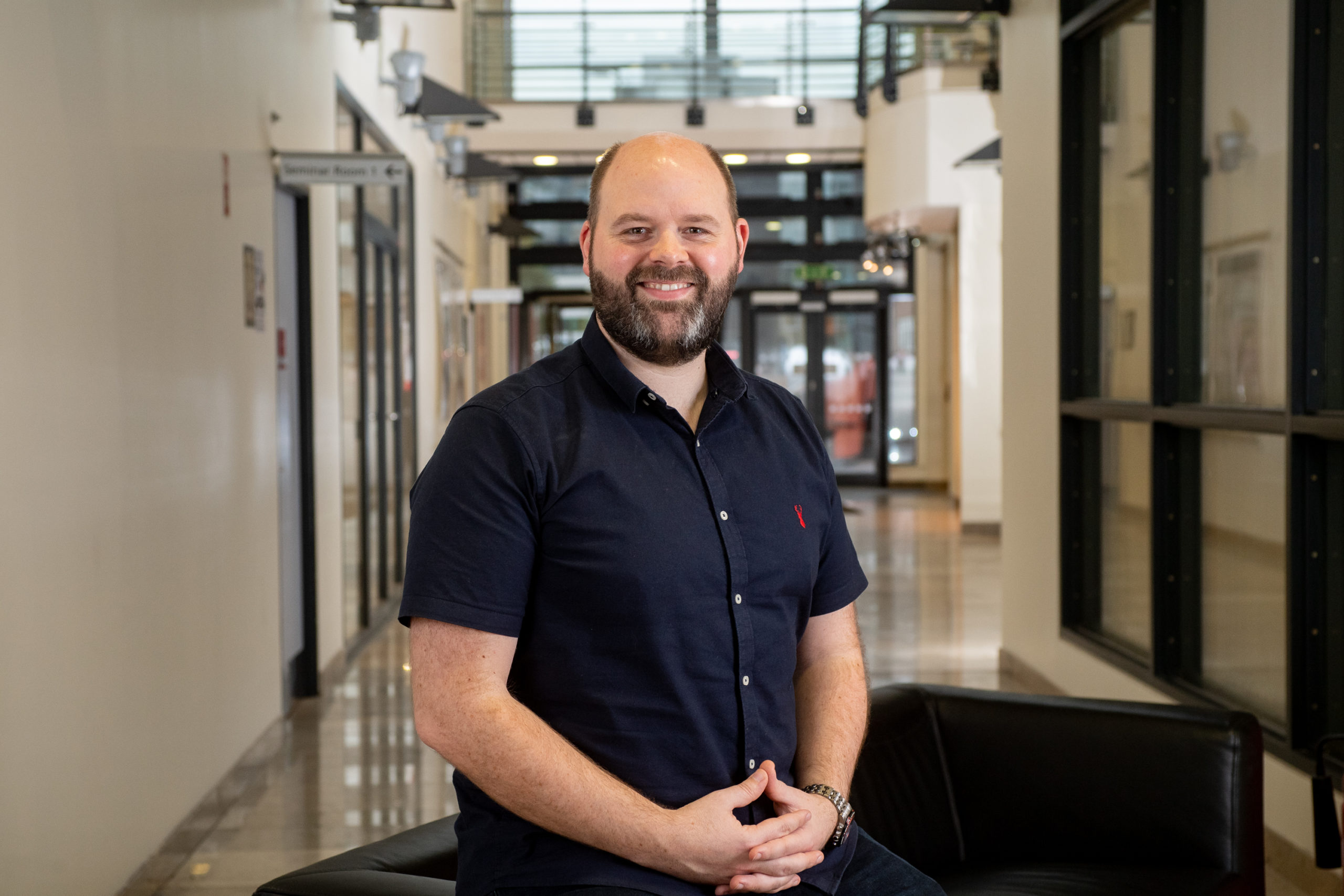
Biography
Matt is the Scientific Lead for Tissue Hybridisation & Digital Pathology in the Precision Medicine Centre of Excellence at Queen’s University Belfast. The TH&DP team’s mission is to deliver clinical trial projects, programmatic grant funded research, and contract research projects for industry.
Prior to this role, Matt was a Post-Doctoral Research Fellow in the Molecular Pathology Group in the Patrick G Johnston Centre for Cancer Research at Queens, and a previous Post-Doctoral Research Fellow at the University of Leeds in the Breast Cancer Research Group in the Leeds Institute of Cancer and Pathology. Matt’s research interests are focused on the digital pathological assessment of immune biomarkers in solid tumours and the application of AI to pathology in both research and a clinical diagnostic settings.
He has published extensively in the field of biomarker validation, multiplexing immunohistochemistry, and digital pathology analysis.
Abstract: Artificial Intelligence In Digital Pathology: A Roadmap To Routine Use In Clinical Practice
Histopathology has undergone major changes, first with the introduction of immunohistochemistry, and latterly with genomic medicine. We argue that a third revolution is underway: artificial intelligence (AI). Coming on the back of digital pathology, the introduction of AI has the potential to both challenge traditional practice and provide a totally new realm for pathology diagnostics. Here, we stress the importance of certified pathologists having learned from the experience of previous revolutions, being willing to accept such disruptive technologies, and being ready to innovate and actively engage in the creation, appl ication and validation of technologies, and oversee the safe introduction of AI into diagnostic practice.
Pathology has experienced the adoption of several major disruptive technologies, beginning with the introduction of immunohistochemistry, and recently with genomic medicine. Suggested to be “the third revolution in pathology”, artificial intelligence (AI) has the potential to challenge the traditional paradigm, especially with regard to routine molecular diagnostic assessment of biomarkers, which can be troublesome and time consuming to evaluate. It is essential that certified pathologists embrace and engage with these disruptive technologies. The advent and adoption of digital pathology is the ideal conduit for the creation and validation of AI applications for diagnostic histopathology practice. The development of robust AI tools requires stringent quality control to rigorous standards. The hybrid nature of our laboratory in the Precision Medicine Centre of Excellence at Queens University Belfast provides an ideal proving ground for AI development, and its application to research and clinical practice. Our state of the art UKAS accredited laboratory houses the Molecular Diagnostic NHS service, compliant to ISO:15189 standards, as well as integrated Genomics, Bioinformatics and Tissue Hybridisation & Digital Pathology groups, where the creation of bespoke AI tools can be generated, tested and applied across a translational environment. We believe our end-to-end workflows; from initial high quality tissue resources, through automated staining and scanning, to specialist pathologist annotation and bespoke deep learning models, represent a stringent roadmap for the generation of AI tools with significant research and clinical applications.
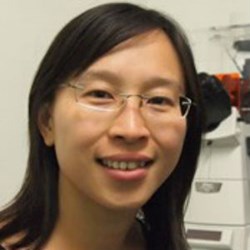
Yinyin Yuan
The Institute Of Cancer Research, London, UK
Biography
Yinyin Yuan leads the Computational Pathology and Integrated Genomics team at the Institute of Cancer Research, London. Building on 15 years of experience in machine learning and 10 years in digital pathology, she aims to deliver practical solutions and machine learning innovations for histopathology and genomics.
Her research focuses on the emerging concept that tumours are complex, evolving ecosystems with dynamic crosstalk between cancer and normal cells. By combining computational pathology and genomics, her team collaborates closely with pathologists, clinicians and biologists to understand how cancer cells evolve genetic diversity and drug resistance within the tumour microenvironment.
Abstract: Reproducible AI for digital pathology: From early detection to cancer treatment
One of the fundamental questions in cancer biology is how cancer evolves. Tumours are complex, evolving ecosystems with dynamic crosstalk among cancer and normal cells. Measuring spatial heterogeneity in the tumour microenvironment is a critical first step towards understanding the spatial context in which cancer develops and evolves. I will discuss quantitative spatial histology measures of immune response as a result of deploying machine learning and spatial statistics widely used in ecology. Geospatial variation in the immune microenvironment can have profound clinical implications, both for early diagnosis and in cancers with low immunogenicity, such as estrogen-receptor positive breast cancer. I will also share our recent progress on studying the geospatial complexity of tumour microenvironment in the TRACERx study of lung cancer evolution. Our AI based image-omics techniques to integrate digital pathology and omics data shed light on the genetics that co-evolves with immune ecological environment to drive immune evasion.
Topics to be covered
- Machine learning and MRI
- Functional imaging, radiomics and big data
- Multiplex immunofluorescence imaging of the tumor microenvironment
- Integrating omics data with digital pathology
- Predicting and understanding tumor progression and treatment response
Who should attend?
- Data analysts dealing with imaging data using deep learning/machine learning
- Clinical and experimental cancer researchers interested in a broad range of imaging modalities including CT, MRI, PET/CT and PET/MR hybrid imaging, as well as optical techniques
- Industry scientists interested in identifying promising solutions for diagnostic and clinical applications
Scientific Program Committee
Thomas C Booth, King’s College London, UK
Carole Lartizien, Université de Lyon, France
Ellen Van Obberghen-Schilling, Université Côte d’Azur, France
ISBI 2021 General Chairs
Laure Blanc-Féraud, CNRS, Université Côte d’Azur, France
Françoise Peyrin, Université de Lyon, France